预测结果融合策略
Voting
- Voting(投票机制)分为软投票和硬投票两种,其原理采用少数服从多数的思想,此方法可用于解决分类问题。
- 硬投票:对多个模型直接进行投票,最终投票数最多的类为最终被预测的类。
- 软投票:和硬投票原理相同,其增加了设置权重的功能,可以为不同模型设置不同权重,进而区分模型不同的重要度。
软投票示例
- 下面以 iris 数据集为例,对模型融合的软投票策略进行说明。代码如下:
import numpy as np
import matplotlib.pyplot as plt
import matplotlib.gridspec as gridspec
import itertools
from sklearn.linear_model import LogisticRegression
from sklearn.svm import SVC
from sklearn.ensemble import RandomForestClassifier
from mlxtend.classifier import EnsembleVoteClassifier
from mlxtend.data import iris_data
from mlxtend.plotting import plot_decision_regions
%matplotlib inline
clf1 = LogisticRegression(random_state=20, solver='lbfgs', multi_class='auto')
clf2 = RandomForestClassifier(random_state=20, n_estimators=100)
clf3 = SVC(random_state=200, probability=True, gamma='auto')
eclf = EnsembleVoteClassifier(clfs=[clf1, clf2, clf3], weights=[2, 1, 1], voting='soft')
X, y = iris_data()
X = X[:, [0, 2]]
gs = gridspec.GridSpec(1, 4)
fig = plt.figure(figsize=(16, 4))
for clf, lab, grd in zip(
[clf1, clf2, clf3, eclf],
['Logistic Regression', 'Random Forest', 'RBF kernel SVM', 'Ensemble'],
itertools.product([0, 1], repeat=2)):
clf.fit(X, y)
ax = plt.subplot(gs[0, grd[0] * 2 + grd[1]])
fig = plot_decision_regions(X=X, y=y, clf=clf, legend=2)
plt.title(lab)
plt.show()
- 运行结果是当采用逻辑回归、随机森林、SVM 模型及模型融合时,数据集的分类预测情况的可视化显示:
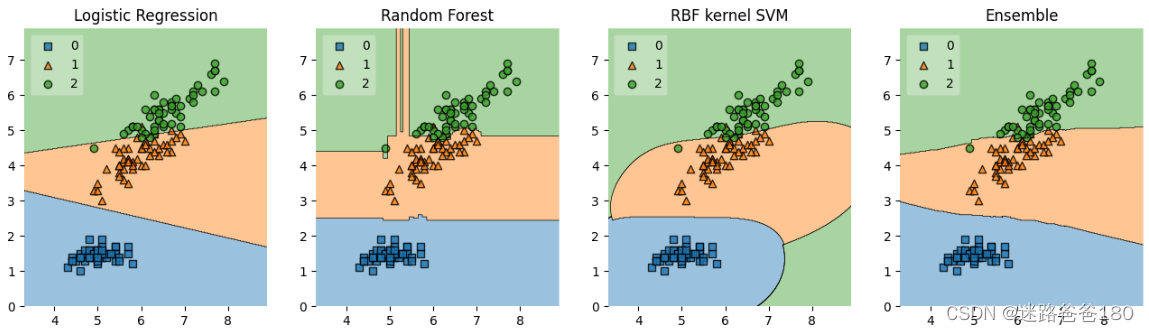