目录
扫描二维码关注公众号,回复:
14838735 查看本文章
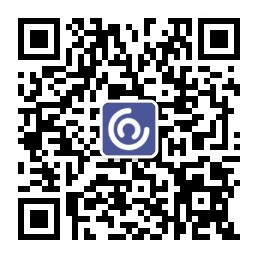
一、分段示例
1.导入必要的库
import numpy as np
import pandas as pd
import matplotlib.pyplot as plt
import seaborn as sns
import sklearn
2.对数据进行初步查看,获取大致信息
可以看到,该文件大小为61878*95,其中最后一列为目标标签;其中还发现该文件数据没有缺失值和重复值。
df=pd.read_csv('C:/Users/27812/Desktop/3-Otto_train.csv')
print(df.info())#发现没有缺失值
print(df.head(5))
print(df.describe())
#发现这个文件的大小为61878*95,其中最后一列预测数据类型为object类型,其余全为float类型
print(df[df.duplicated()])#查看是否有重复值,此时返回一个[],无重复值
3.编码
最后一列是object类型,这里采用字典编码进行转换。
#将最后一列的target进行编码,转换成数值
#这里采用字典编码,注意:对应的object分类值变成0,1,2...
def change_object_cols(se):
value=se.unique().tolist()
value.sort()
return se.map(pd.Series(range(len(value)),index=value)).values
df['target']=change_object_cols(df['target'])
4.查看数值是否重复或唯一
#查看每列数值是否唯一
all_cloumns=list(df)
print(all_cloumns)#以列表形式返回所有列名
print(df.nunique())#粗略查看每列唯一值数量
for i in all_cloumns[0:96]:
print(df[i].nunique())#详细查看每列唯一值的数量,发现id列只是个计数列,target总共有九种
5.数据可视化
相关可视化效果展示如下
#数据可视化
#首先查看最后一列的分布情况
diction=dict(df['target'].value_counts())#统计不同分类结果的数量
print(diction)
#{1: 16122, 5: 14135, 7: 8464, 2: 8004, 8: 4955, 6: 2839, 4: 2739, 3: 2691, 0: 1929}
values=diction.values()
label=diction.keys()
plt.pie(values,labels=label,autopct='%10.1f%%')#绘制饼图
plt.show()
plt.savefig('C:/Users/27812/Desktop/a.png',dpi=300)
#我们发现,类型1和类型5所占比重很大
#这里采用seaborn库来可视化
#因为特征很多,有93个特征和9个分类值,所以这里抽取部分特征进行可视化
df_1=df.loc[200:400,:]#选取前4个特征值对应的200行数据进行可视化
pairplot_fig1=sns.pairplot(df_1,kind='reg',
diag_kind='hist',
hue='target',
vars=['feat_1', 'feat_2', 'feat_3', 'feat_4'])
fig_path1='C:/Users/27812/Desktop/图片1'
pairplot_fig1.savefig(fig_path1,dpi=500)#保存图片
#选取前4个特征对应的所有行数据进行可视化
pairplot_fig2=sns.pairplot(df,kind='reg',
diag_kind='hist',
hue='target',
vars=['feat_1', 'feat_2', 'feat_3', 'feat_4'])
fig_path1='C:/Users/27812/Desktop/图片2'
pairplot_fig2.savefig(fig_path1,dpi=500)#保存图片
jointplot_fig3=sns.jointplot(x='feat_42',y='target',data=df,kind='kde')
fig_path2='C:/Users/27812/Desktop/图片3'
jointplot_fig3.savefig(fig_path2,dpi=500)#保存图片
6.划分数据集
'''
#注:决策树不是基于距离计算的算法模型,所以不需要归一化
'''
#划分数据集
from sklearn.model_selection import train_test_split
x_train,x_test,y_train,y_test = train_test_split(df[all_cloumns[1:95]],
df[all_cloumns[-1]],
test_size=0.3)
7.运用决策树进行分类
#导入
from sklearn import tree
clf = tree.DecisionTreeClassifier(criterion='gini',#选择基尼系数
random_state=42,
splitter='random',
max_depth=30,
min_samples_leaf=10,
min_samples_split=10) #实例化
clf = clf.fit(x_train,y_train) #训练
result = clf.score(x_test,y_test) #为测试集打分
print('测试集打分',result)
print('训练集打分',clf.score(x_train,y_train))
'''
测试集打分 0.9995690583925878
训练集打分 0.9995151683058595
'''
二、完整代码
import numpy as np
import pandas as pd
import matplotlib.pyplot as plt
import seaborn as sns
import sklearn
df=pd.read_csv('C:/Users/27812/Desktop/3-Otto_train.csv')
print(df.info())#发现没有缺失值
print(df.head(5))
print(df.describe())
#发现这个文件的大小为61878*95,其中最后一列预测数据类型为object类型,其余全为float类型
print(df[df.duplicated()])#查看是否有重复值,此时返回一个[],无重复值
#将最后一列的target进行编码,转换成数值
#这里采用字典编码,注意:对应的object分类值变成0,1,2...
def change_object_cols(se):
value=se.unique().tolist()
value.sort()
return se.map(pd.Series(range(len(value)),index=value)).values
df['target']=change_object_cols(df['target'])
#查看每列数值是否唯一
all_cloumns=list(df)
print(all_cloumns)#以列表形式返回所有列名
print(df.nunique())#粗略查看每列唯一值数量
for i in all_cloumns[0:96]:
print(df[i].nunique())#详细查看每列唯一值的数量,发现id列只是个计数列,target总共有九种
#数据可视化
#首先查看最后一列的分布情况
diction=dict(df['target'].value_counts())#统计不同分类结果的数量
print(diction)
#{1: 16122, 5: 14135, 7: 8464, 2: 8004, 8: 4955, 6: 2839, 4: 2739, 3: 2691, 0: 1929}
values=diction.values()
label=diction.keys()
plt.pie(values,labels=label,autopct='%10.1f%%')#绘制饼图
plt.show()
plt.savefig('C:/Users/27812/Desktop/a.png',dpi=300)
#我们发现,类型1和类型5所占比重很大
#这里采用seaborn库来可视化
#因为特征很多,有93个特征和9个分类值,所以这里抽取部分特征进行可视化
df_1=df.loc[200:400,:]#选取前4个特征值对应的200行数据进行可视化
pairplot_fig1=sns.pairplot(df_1,kind='reg',
diag_kind='hist',
hue='target',
vars=['feat_1', 'feat_2', 'feat_3', 'feat_4'])
fig_path1='C:/Users/27812/Desktop/图片1'
pairplot_fig1.savefig(fig_path1,dpi=500)#保存图片
#选取前4个特征对应的所有行数据进行可视化
pairplot_fig2=sns.pairplot(df,kind='reg',
diag_kind='hist',
hue='target',
vars=['feat_1', 'feat_2', 'feat_3', 'feat_4'])
fig_path1='C:/Users/27812/Desktop/图片2'
pairplot_fig2.savefig(fig_path1,dpi=500)#保存图片
jointplot_fig3=sns.jointplot(x='feat_42',y='target',data=df,kind='kde')
fig_path2='C:/Users/27812/Desktop/图片3'
jointplot_fig3.savefig(fig_path2,dpi=500)#保存图片
'''
#注:决策树不是基于距离计算的算法模型,所以不需要归一化
'''
#划分数据集
from sklearn.model_selection import train_test_split
x_train,x_test,y_train,y_test = train_test_split(df[all_cloumns[1:95]],
df[all_cloumns[-1]],
test_size=0.3)
#导入
from sklearn import tree
clf = tree.DecisionTreeClassifier(criterion='gini',#选择基尼系数
random_state=42,
splitter='random',
max_depth=30,
min_samples_leaf=10,
min_samples_split=10) #实例化
clf = clf.fit(x_train,y_train) #训练
result = clf.score(x_test,y_test) #为测试集打分
print('测试集打分',result)
print('训练集打分',clf.score(x_train,y_train))
'''
测试集打分 0.9995690583925878
训练集打分 0.9995151683058595
'''