LLMs之Baichuan:Baichuan-13B模型的简介(包括Baichuan-7B)、安装、使用方法之详细攻略
导读:2023年6月15日,百川智能(搜狗创始人王小川创建)发布了首个大模型成果Baichuan-7B,开源可商用,它在C-Eval、AGIEval和Gaokao中文权威评测榜单上,以显著优势全面超过了ChatGLM-6B等其他大模型,并且在MMLU英文权威评测榜单上,也领先LLaMA-7B很多。
要来就来大的,要玩就玩真的,人狠话不多,就在7月11日,百川智能发布包含有预训练 (Baichuan-13B-Base) 和对齐 (Baichuan-13B-Chat) 两个版本。
分析Baichuan-13B 的技术:Baichuan-13B 整体模型基于标准的 Transformer 结构,并在高质量的语料上(词表为64K)训练了 1.4 万亿 tokens,上下文窗口长度为 4096。Baichuan-13B 采样ALiBi 线性偏置技术提高了推理性能,但是早先的Baichuan-7B采样的是RoPE。它还支持 int8 和 int4 量化,其中int4仅需9.7 GPU显存,当然,也支持CPU推理但需要 60GB 内存。
分析Baichuan-7B的技术:位置编码采用rotary-embedding,激活函数采用SwiGLU,基于RMSNorm 的 Pre-Normalization,并采用 DeepSpeed 框架进行训练,工程上采用了包括算子优化、算子切分、混合精度、训练容灾、通信优化的五大技术增速提效。
>> 算子优化技术:采用更高效算子,如 Flash-attention,NVIDIA apex 的 RMSNorm 等。
>> 算子切分技术:将部分计算算子进行切分,减小内存峰值。
>> 混合精度技术:降低在不损失模型精度的情况下加速计算过程。
>> 训练容灾技术:训练平台和训练框架联合优化,IaaS + PaaS 实现分钟级的故障定位和任务恢复。
>> 通信优化技术,具体包括:采用拓扑感知的集合通信算法,避免网络拥塞问题,提高通信效率;根据卡数自适应设置 bucket size,提高带宽利用率;根据模型和集群环境,调优通信原语的触发时机,从而将计算和通信重叠。---------------------------------------------------------------------------------
近一段时间,不论是从外部公开发布,还是与内部各位业界大佬在线上探讨或者私下交流时,博主深深地感觉到,太卷了,大模型领域是贼卷啊,周围的业界人士,都在把玩各种tricks,博主本人是深深的感受到了……国内AI公司,不出来弄个开源模型,总感觉自己啥都不是啊……路人甲:啥?你是搞AIGC的?别先说话,你先告诉我你是几B的?有多少亿的token?你是哪个style?用的是SwiGLU吧,RoPE吧,还有个Flash Attention,加点AMP,给个4-bit,你说!你到底有没有用Fast?ComOpt呢?有木有AimOpt?哎,你倒是,你说话呀?
目录
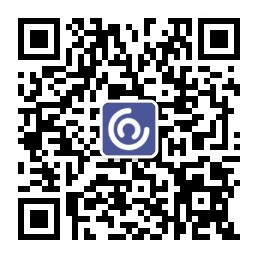
Baichuan-13B模型的简介
Baichuan-13B 是由百川智能继 Baichuan-7B 之后开发的包含 130 亿参数的开源可商用的大规模语言模型,在权威的中文和英文 benchmark 上均取得同尺寸最好的效果。本次发布包含有预训练 (Baichuan-13B-Base) 和对齐 (Baichuan-13B-Chat) 两个版本。Baichuan-13B 有如下几个特点:
- 更大尺寸、更多数据:Baichuan-13B 在 Baichuan-7B 的基础上进一步扩大参数量到 130 亿,并且在高质量的语料上训练了 1.4 万亿 tokens,超过 LLaMA-13B 40%,是当前开源 13B 尺寸下训练数据量最多的模型。支持中英双语,使用 ALiBi 位置编码,上下文窗口长度为 4096。
- 同时开源预训练和对齐模型:预训练模型是适用开发者的『 基座 』,而广大普通用户对有对话功能的对齐模型具有更强的需求。因此本次开源我们同时发布了对齐模型(Baichuan-13B-Chat),具有很强的对话能力,开箱即用,几行代码即可简单的部署。
- 更高效的推理:为了支持更广大用户的使用,我们本次同时开源了 int8 和 int4 的量化版本,相对非量化版本在几乎没有效果损失的情况下大大降低了部署的机器资源门槛,可以部署在如 Nvidia 3090 这样的消费级显卡上。
- 开源免费可商用:Baichuan-13B 不仅对学术研究完全开放,开发者也仅需邮件申请并获得官方商用许可后,即可以免费商用。
GitHub地址:https://github.com/baichuan-inc/Baichuan-13B
1、测试结果——Benchmark结果
我们在各个权威大语言模型的中英文 benchmark 上进行了5-shot
评测。结果如下:
C-Eval
Model 5-shot | STEM | Social Sciences | Humanities | Others | Average |
---|---|---|---|---|---|
Baichuan-7B | 38.2 | 52.0 | 46.2 | 39.3 | 42.8 |
Chinese-Alpaca-Plus-13B | 35.2 | 45.6 | 40.0 | 38.2 | 38.8 |
Vicuna-13B | 30.5 | 38.2 | 32.5 | 32.5 | 32.8 |
Chinese-LLaMA-Plus-13B | 30.3 | 38.0 | 32.9 | 29.1 | 32.1 |
Ziya-LLaMA-13B-Pretrain | 27.6 | 34.4 | 32.0 | 28.6 | 30.0 |
LLaMA-13B | 27.0 | 33.6 | 27.7 | 27.6 | 28.5 |
moss-moon-003-base (16B) | 27.0 | 29.1 | 27.2 | 26.9 | 27.4 |
Baichuan-13B-Base | 45.9 | 63.5 | 57.2 | 49.3 | 52.4 |
Baichuan-13B-Chat | 43.7 | 64.6 | 56.2 | 49.2 | 51.5 |
MMLU
Model 5-shot | STEM | Social Sciences | Humanities | Others | Average |
---|---|---|---|---|---|
Vicuna-13B | 40.4 | 60.5 | 49.5 | 58.4 | 52.0 |
LLaMA-13B | 36.1 | 53.0 | 44.0 | 52.8 | 46.3 |
Chinese-Alpaca-Plus-13B | 36.9 | 48.9 | 40.5 | 50.5 | 43.9 |
Ziya-LLaMA-13B-Pretrain | 35.6 | 47.6 | 40.1 | 49.4 | 42.9 |
Baichuan-7B | 35.6 | 48.9 | 38.4 | 48.1 | 42.3 |
Chinese-LLaMA-Plus-13B | 33.1 | 42.8 | 37.0 | 44.6 | 39.2 |
moss-moon-003-base (16B) | 22.4 | 22.8 | 24.2 | 24.4 | 23.6 |
Baichuan-13B-Base | 41.6 | 60.9 | 47.4 | 58.5 | 51.6 |
Baichuan-13B-Chat | 40.9 | 60.9 | 48.8 | 59.0 | 52.1 |
说明:我们采用了 MMLU 官方的评测方案。
CMMLU
Model 5-shot | STEM | Humanities | Social Sciences | Others | China Specific | Average |
---|---|---|---|---|---|---|
Baichuan-7B | 34.4 | 47.5 | 47.6 | 46.6 | 44.3 | 44.0 |
Vicuna-13B | 31.8 | 36.2 | 37.6 | 39.5 | 34.3 | 36.3 |
Chinese-Alpaca-Plus-13B | 29.8 | 33.4 | 33.2 | 37.9 | 32.1 | 33.4 |
Chinese-LLaMA-Plus-13B | 28.1 | 33.1 | 35.4 | 35.1 | 33.5 | 33.0 |
Ziya-LLaMA-13B-Pretrain | 29.0 | 30.7 | 33.8 | 34.4 | 31.9 | 32.1 |
LLaMA-13B | 29.2 | 30.8 | 31.6 | 33.0 | 30.5 | 31.2 |
moss-moon-003-base (16B) | 27.2 | 30.4 | 28.8 | 32.6 | 28.7 | 29.6 |
Baichuan-13B-Base | 41.7 | 61.1 | 59.8 | 59.0 | 56.4 | 55.3 |
Baichuan-13B-Chat | 42.8 | 62.6 | 59.7 | 59.0 | 56.1 | 55.8 |
说明:CMMLU 是一个综合性的中文评估基准,专门用于评估语言模型在中文语境下的知识和推理能力。我们采用了其官方的评测方案。
2、模型细节
模型名称 | 隐藏层维度 | 层数 | 注意力头数 | 词表大小 | 总参数量 | 训练数据(tokens) | 位置编码 | 最大长度 |
---|---|---|---|---|---|---|---|---|
Baichuan-7B | 4,096 | 32 | 32 | 64,000 | 7,000,559,616 | 1.2 万亿 | RoPE | 4,096 |
Baichuan-13B | 5,120 | 40 | 40 | 64,000 | 13,264,901,120 | 1.4 万亿 | ALiBi | 4,096 |
Baichuan-13B模型的安装
baichuan-inc/Baichuan-13B-Base下载
https://huggingface.co/baichuan-inc/Baichuan-13B-Base
baichuan-inc/Baichuan-13B-Chat下载
baichuan-inc/Baichuan-13B-Chat · Hugging Face
Baichuan-13B模型的使用方法
1、推理和部署
推理所需的模型权重、源码、配置已发布在 Hugging Face:Baichuan-13B-Base 和 Baichuan-13B-Chat。下面以 Baichuan-13B-Chat 为例示范多种推理方式。程序会自动从 Hugging Face 下载所需资源。
推理前请安装依赖:
pip install -r requirements.txt
T1、Python代码方式
>>> import torch
>>> from transformers import AutoModelForCausalLM, AutoTokenizer
>>> from transformers.generation.utils import GenerationConfig
>>> tokenizer = AutoTokenizer.from_pretrained("baichuan-inc/Baichuan-13B-Chat", use_fast=False, trust_remote_code=True)
>>> model = AutoModelForCausalLM.from_pretrained("baichuan-inc/Baichuan-13B-Chat", device_map="auto", torch_dtype=torch.float16, trust_remote_code=True)
>>> model.generation_config = GenerationConfig.from_pretrained("baichuan-inc/Baichuan-13B-Chat")
>>> messages = []
>>> messages.append({"role": "user", "content": "世界上第二高的山峰是哪座"})
>>> response = model.chat(tokenizer, messages)
>>> print(response)
乔戈里峰。世界第二高峰———乔戈里峰西方登山者称其为k2峰,海拔高度是8611米,位于喀喇昆仑山脉的中巴边境上
T2、命令行工具方式
python cli_demo.py
最后输出示例如下:
T3、网页 demo 方式
依靠streamlit运行以下命令,会在本地启动一个 web 服务,把控制台给出的地址放入浏览器即可访问。
streamlit run web_demo.py
效果如下:
2、推理性能
Baichuan-13B 使用了 ALiBi 线性偏置技术,相对于 Rotary Embedding 计算量更小,对推理性能有显著提升;与标准的 LLaMA-13B 相比,平均推理速度 (tokens/s) 实测提升 31.6%:
Model | tokens/s |
---|---|
LLaMA-13B | 19.4 |
Baichuan-13B | 25.4 |
测试环境和参数:GPU A100-SXM4-80G, PyTorch 2.0.0+cu117, transformers 4.29.1, batch size = 1, 生成长度 = 2048, 精度 fp16, 基于 Baichuan-13B-Base
3、量化部署
Baichuan-13B 支持 int8 和 int4 量化,用户只需在推理代码中简单修改两行即可实现。请注意,如果是为了节省显存而进行量化,应加载原始精度模型到 CPU 后再开始量化;避免在from_pretrained
时添加device_map='auto'
或者其它会导致把原始精度模型直接加载到 GPU 的行为的参数。
如需使用 int8 量化:
model = AutoModelForCausalLM.from_pretrained("baichuan-inc/Baichuan-13B-Chat", torch_dtype=torch.float16, trust_remote_code=True)
model = model.quantize(8).cuda()
同样的,如需使用 int4 量化:
model = AutoModelForCausalLM.from_pretrained("baichuan-inc/Baichuan-13B-Chat", torch_dtype=torch.float16, trust_remote_code=True)
model = model.quantize(4).cuda()
另外,如果你不想调用 quantize 在线量化,我们有量化好的 int8 Chat 模型可供使用:Baichuan-13B-Chat-int8:
model = AutoModelForCausalLM.from_pretrained("baichuan-inc/Baichuan-13B-Chat-int8", torch_dtype=torch.float16, trust_remote_code=True).cuda()
量化前后占用显存情况如下:
Precision | GPU Mem (GB) |
---|---|
bf16 / fp16 | 26.0 |
int8 | 15.8 |
int4 | 9.7 |
量化后在各个 benchmark 上的结果和原始版本对比如下:
Model 5-shot | C-Eval | MMLU | CMMLU |
---|---|---|---|
Baichuan-13B-Base | 52.4 | 51.6 | 55.3 |
Baichuan-13B-Base-int8 | 51.2 | 49.9 | 54.5 |
Baichuan-13B-Base-int4 | 47.6 | 46.0 | 51.0 |
4、CPU 部署
Baichuan-13B 支持 CPU 推理,但需要强调的是,CPU 的推理速度相对较慢。需按如下方式修改模型加载的方式:
model = AutoModelForCausalLM.from_pretrained("baichuan-inc/Baichuan-13B-Chat", torch_dtype=torch.float32, trust_remote_code=True)
使用CPU进行推理大概需要 60GB 内存。